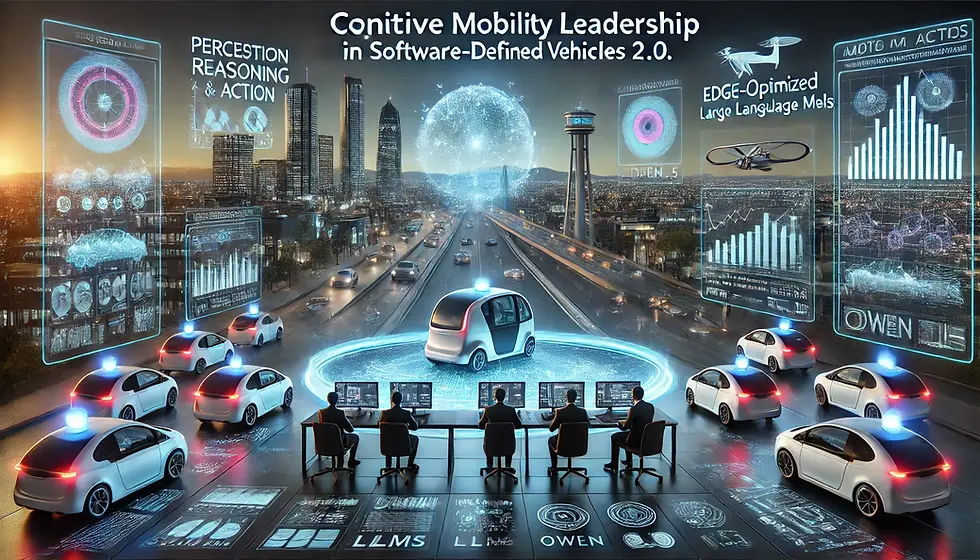
February 3, 2025
The automotive and air mobility industries are undergoing a paradigm shift. Software-defined vehicles (SDVs) are evolving from connected machines into cognitive ecosystems, powered by AI architectures that fuse perception, reasoning, and action. For executives and decision-makers, edge-optimized large language models (LLMs) like DeepSeek R1 and OWen 2.5 aren’t just technical tools—they’re strategic differentiators that redefine safety, customer value, and operational efficiency in the age of autonomy.
To stay ahead, leaders must address two critical challenges:
Data Privacy: How to train and deploy LLMs without compromising sensitive user or operational data.
Edge-Cloud Continuum: Balancing real-time processing with scalable cloud intelligence.
Here’s how federated learning and edge-optimized LLMs unlock this future while aligning with your organization’s strategic roadmap.
Why Edge LLMs Matter: A Leadership Perspective
Edge LLMs are game-changers for SDV 2.0:
Safety: Contextualize sensor data into actionable insights (e.g., predicting cyclist intent in urban mobility corridors).
Efficiency: Enable predictive maintenance, reducing downtime for autonomous fleets by 30% (see my analysis on transforming software-defined vehicles).
Experience: Deliver voice-driven interfaces that learn driver preferences across ground and aerial vehicles.
The stakes? Companies that master edge LLMs will dominate high-margin segments like autonomous ride-hailing and smart logistics.
Federated Learning & the Edge-Cloud Continuum: Privacy by Design
One of the biggest barriers to LLM adoption is data privacy. Federated learning (FL) solves this by training models across distributed devices without sharing raw data.
LLMs for the Edge-Cloud Continuum
DeepSeek R1: Optimized for edge deployment, it supports federated learning workflows to ensure privacy-compliant AI training (learn more in my Edge AI comparison).
OWen 2.5: Processes sensor data locally while using FL to aggregate anonymized model updates across fleets.
Microsoft Phi-3: A compact LLM that runs on-device with FL support for cross-vehicle knowledge sharing.
How It Works:
Local Inference: LLMs process data on-device (e.g., in a car or eVTOL).
Federated Updates: Only encrypted model updates (not raw data) are sent to the cloud.
Global Refinement: Aggregated models improve all devices while complying with GDPR/CCPA.
This approach mirrors the principles I outlined in Redefining AI Computing Power at the Edge, where edge intelligence meets scalable cloud coordination.
Strategic Playbook for SDV 2.0 Leaders
1. Build Ecosystem Alliances
AI Startups + Tier 1s: Qualcomm’s partnership with Stability AI to compress LLMs for Snapdragon Ride Flex SoCs.
Cross-Industry Data Pools: Hyundai’s Supernal (air mobility) and Motional (autonomous driving) share FL frameworks for multi-domain learning.
2. Prioritize Modular Architectures
Software-Defined Hardware: Adopt adaptive platforms like Qualcomm’s SA9000P, which scales from L2+ to L4 autonomy.
Edge-Cloud Hybridization: Use 5G to offload non-safety LLM tasks (e.g., voice assistants) while keeping safety-critical processing local.
3. Navigate Regulatory Complexity
Proactive Compliance: Align with ISO 21448 (SOTIF) addresses the Safety of the Intended Functionality for autonomous systems and UL 4600 a safety standard for autonomous vehicles that evaluates AI-driven systems to certify probabilistic LLM outputs.
Global Standards Leadership: Shape EU AI Act and FAA rules via consortiums like AVCC (Autonomous Vehicle Computing Consortium) or CAMAA (China Automotive Mobility Alliance).
Why They Matter:
SOTIF + UL 4600: Provide frameworks to certify AI systems where traditional safety standards (ISO 26262) fall short.
AVCC + CAMAA: Enable global interoperability (AVCC) and regional scalability (CAMAA) for autonomous technologies.
Case Studies: Federated Learning in Action
Bosch’s AI ECU
Strategy: Deployed FL to train LLMs across 500k vehicles without accessing raw driver data.
Result: Faster anomaly detection in braking systems.
Archer Aviation’s Midnight eVTOL
Edge LLM Use Case: FL-trained models negotiate airspace with FAA systems during urban flights.
Key Insight: Partner with regulators early—Archer collaborates with NASA on AI air traffic protocols.
Mercedes-Benz’s MB.OS
Vision: Unified OS for cars and aerial vehicles, using FL to share anonymized insights across fleets.
Execution: Decoupled software from hardware lifecycles via a $1B+ R&D investment.
Risks & Mitigation: A C-Suite Lens
Tech Debt: Avoid vendor lock-in via open-source FL frameworks like TensorFlow Federated.
Talent Gaps: Acquire MLOps expertise for edge deployment—GM’s Cruise acquisition prioritized FL engineers.
Cybersecurity: Deploy hardware-rooted trust zones (e.g., AMD’s Secure Processor) for model integrity.
The Future: Unified Mobility Ecosystems
By 2030, edge LLMs will enable cognitive networks where cars, drones, and eVTOLs collaborate via shared AI layers. Imagine:
A delivery drone re-routing due to weather, with its LLM advising connected trucks to adjust routes.
An autonomous taxi negotiating priority at a 4D intersection via LLM-mediated V2X communication.
Call to Action: Lead the Cognitive Revolution
Audit Capabilities: Map your AI stack against edge LLM use cases (see my Edge AI framework).
Invest Boldly: Dedicate 15-20% of R&D budgets to edge-cloud AI infrastructure.
Collaborate to Scale: Join forces with regulators and competitors to standardize FL workflows.
The winners of SDV 2.0 won’t just adopt edge LLMs—they’ll architect the cognitive future of mobility.
Further Reading:
Comments